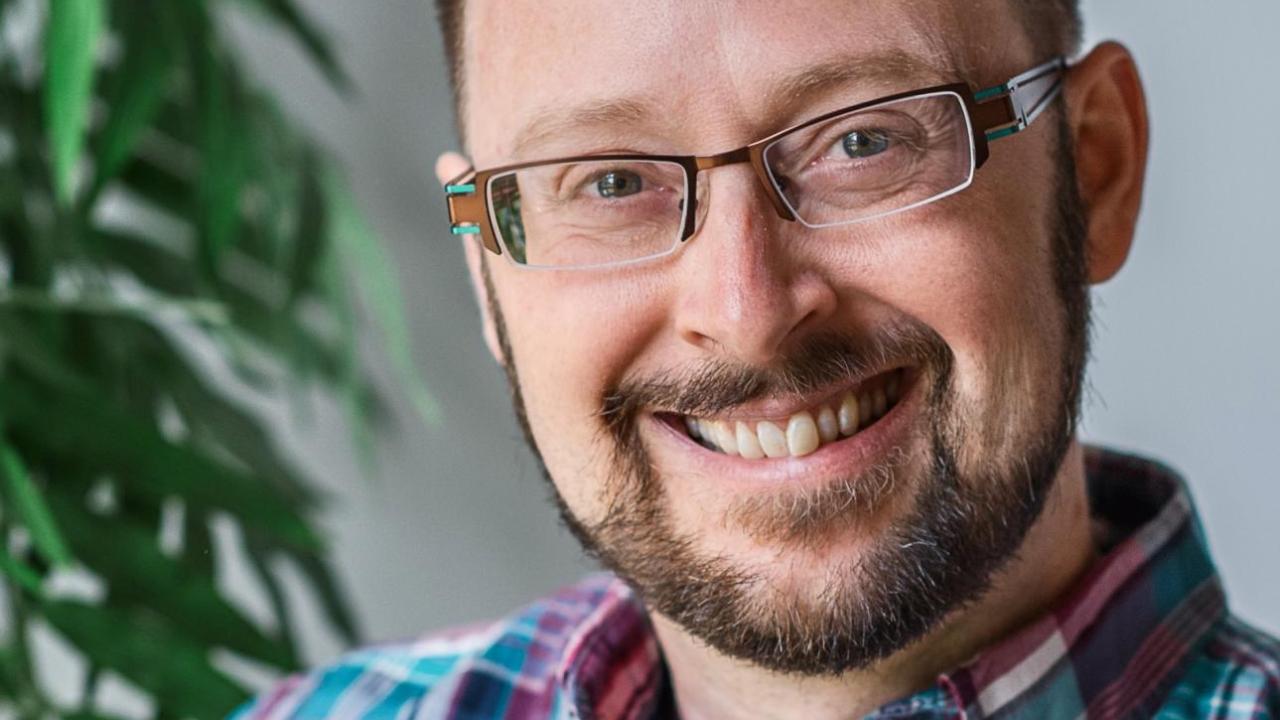
Event Date
A recording of this presentation is available here.
PATRICK PILARSKI, PHD
Canada CIFAR Artificial Intelligence Chair
Fellow & Board of Directors, Alberta Machine Intelligence Institute
Associate Professor, Division of Physical Medicine and Rehabilitation, Department of Medicine
University of Alberta
Host: Jonathon Schofield, PhD, jschofield@ucdavis.edu
This is an in-person event. Please register here: https://tinyurl.com/Neuroeng-June2024
Registration for remote attendance is limited to colleagues from the Sacramento campus and those working remotely: https://tinyurl.com/NeuroengJun24
Abstract
As powered upper-limb prostheses have advanced through technological innovation, new surgical capabilities, and enhanced clinical care and training paradigms, their degree of integration with their human users and impact on the training of their human users has changed dramatically. In particular, and as a focus of this seminar, tighter coupling of prostheses with users combined with a rapid step-change in computing capabilities available to prosthesis designers has made it now possible for prosthetic systems to adapt during both training and deployment; more strongly, modern prosthetic technology has the necessary preconditions to construct many key elements of its operation from experience obtained during deployed interactions with users over extended periods of time. Is this a good thing? This talk will introduce the use of artificial intelligence and machine learning methods to continually improve the control of and feedback from myoelectric prostheses, and improve participants’ depth of understanding on these technologies by way of a series of concrete examples drawn from our
past 10 years of work on developing upper-limb prosthetic interfaces (specifically techniques and viewpoints from the field of reinforcement learning). One main outcome of this talk will be increased opportunities and shared language for further dialogue that help our community as a whole better pursue the design of next-generation prosthetic interventions and other rehabilitation technologies.
Bio
Dr. Patrick M. Pilarski is a Canada CIFAR Artificial Intelligence Chair, past Canada Research Chair in Machine Intelligence for Rehabilitation, and an Associate Professor in the Division of Physical Medicine and Rehabilitation, Department of Medicine, University of Alberta. In 2017, Dr. Pilarski co-founded DeepMind's first international research office, located in Edmonton, Alberta, where he served as office co-lead and a Senior Staff Research Scientist until 2023. He is a Fellow and Board of Directors member with the Alberta Machine Intelligence Institute (Amii), co-leads the Bionic Limbs for Improved Natural Control (BLINC) Laboratory, and is a principal investigator with the Reinforcement Learning and Artificial Intelligence Laboratory (RLAI) and the Sensory Motor Adaptive Rehabilitation Technology (SMART) Network at the University of Alberta. Dr. Pilarski received the B.ASc. in Electrical Engineering from the University of British Columbia in 2004, the Ph.D. in Electrical and Computer Engineering from the University of Alberta in 2009, and completed his postdoctoral training in computing science with Dr. Richard S. Sutton at the University of Alberta. Dr. Pilarski's research interests include reinforcement learning and decision making, artificial intelligence, real-time machine learning, human-machine interaction, intelligence amplification, rehabilitation technology, and assistive robotics. He leads the Amii Adaptive Prosthetics Program—an interdisciplinary initiative focused on creating intelligent artificial limbs to restore and extend abilities for people with amputations. As part of this research, Dr. Pilarski has developed and made prominent machine learning techniques for continual sensorimotor control and prediction learning on prosthetic devices. These include some of the first published approaches to ongoing user training of upper-limb prosthesis control systems via reinforcement learning, and he pioneered the use of general value functions in prediction learning to continually adapt myoelectric control interfaces in real time. Dr. Pilarski's research programme continues to explore human-device interaction and communication, long-term co-adaptation and joint action between agents, patient-specific device optimization, and constructivism in tightly coupled human-machine interfaces. He has also created techniques for rapid cancer and pathogen screening through work on biomedical pattern recognition, robotic micro-manipulation of medical samples, and hand-held diagnostic devices. Dr. Pilarski is the award-winning author or co-author of more than 120 peer-reviewed articles, a Senior Member of the IEEE, and has been supported by provincial, national, and international research grants.